Understanding Churn and Effective Methods for Predicting Customer Churn
- Reading Time: 11 minutes
There are issues with client turnover that arise during the life cycle of any organization. However, it is crucial to realize that keeping current or long-standing customers is always easier and less expensive than seeking alternative ones. Consequently, a business should always look for novel ways to increase client loyalty and recognize hazards and signs to prevent revenue losses.
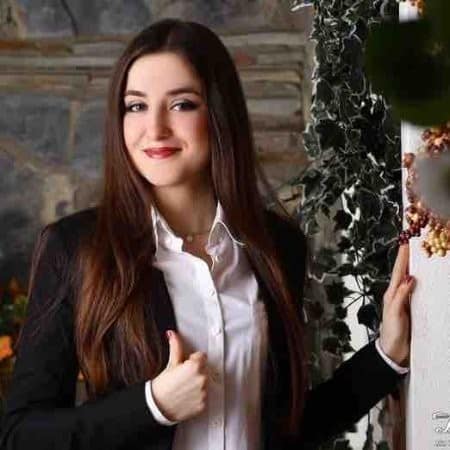
What is churn rate?
The act of determining the overall number of customers during a given period is challenging. Generally, the number of total consumers for a particular month is not well-defined since it might fluctuate over the month as a result of cancellations from the present user base and the onboarding of new customers.
The percentage of customers that have terminated their subscriptions or uninstalled an application during a predetermined time period is known as the churn rate. For subscription based businesses, the most contract payments are made on a regular basis, the attrition has been most frequently quantified as a monthly ratio.
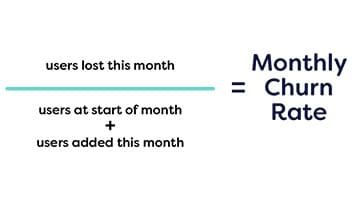
Figure 1: The formula for churn rate
What are the indications of churn?
Even though assessing termination levels is crucial, there are a number of churn indicators you can use to identify customers that are less likely to be renewed. The churn tendency patterns of customers can be detected by observing the most prevalent ones, as described in more detail in the following.
Product utilization patterns
When a customer’s usage rate decreases, it’s typically a sign that attrition is likely to occur. While there are several usage indications, such as the total number of users, user log-ins, and user time spent on the product, turnover is the next step if you observe that a customer’s usage pattern has suddenly changed.
Client interaction usages
Tracking particular metrics is important. They may refer to some signs of future churn patterns if customers are utilizing the website or service less. If a customer’s usage pattern has sharply changed, churn is frequently the next step. Many indicators will differ, such as the steps below:
- Reduced time spent on the website more people who have payments that are past due, more consumers who downgrade their services
- Fewer support tickets are being sent through customer care channels.
- Users are less likely to engage if they don’t make an effort to remedy issues with their product experience.
Price level discontent
The fluctuating service costs present certain challenges for particular businesses. The firms should value the items appropriately if other businesses in the industry lower their pricing to avoid churn behavior.
Customers are generally aware of their choices because they frequently compare prices. Most software subscribers are motivated by cost. No matter how much a consumer values the service or product you offer, if it is unaffordable, many will pick a less expensive alternative.
Sign up to drive your business with the power of data
What is churn prediction?
Churn prediction is the process of identifying consumers who are most likely to leave your business or end a product subscription model based on their usage of the product.
In order to properly forecast attrition, the combination and usage of the key signs that the team has identified are crucial. This will let the business know when a client is likely to leave so that it can take appropriate action.
Why is churn prediction important?
A corporation’s overall performance may be significantly impacted by improved client retention. If a business has a low customer retention rate, even if it is adding new customers every month, it continues to lose out on a huge amount of potential money.
Although many businesses concentrate on expanding their client base and developing plans to save costs associated with customer acquisition, the expense of acquiring a new customer is far higher than the cost of maintaining an existing one is highlighted on a research done by Frederick Reichheld of Bain & Company.
Once the customers are pinpointed who are most likely to cancel, the companies should be able to precisely plan each client’s marketing strategy to increase the likelihood that the client will stay.
Businesses may prevent churn by taking preventative measures when they can anticipate it before it occurs. These measures can be collected in four categories:
- The customers who have tendency to attrite can be reached to offer assistance or assess any unmet needs
- Delivering more focused re-engagement initiatives, such as email reminders
- Developing more targeted client educational resources
- Reassessing bigger corporate retention programs
In addition to identifying at-risk consumers, churn prediction also identifies the pain points that precede customer loss, assisting in boosting overall customer happiness and retention. Churn can be avoided by foreseeing it. As a result, avoiding churn allows companies to find remarkable ways to generate income.
How to generate a churn prediction model?
As a component of churn prevention, the churn approach analyzes users who are in danger of leaving as early as possible. Models for predicting churn are difficult to develop. When customers stop making purchases of products and services, online firms consider them to be “churning.” Sector variables can affect customer turnover, although some frequent causes include the underuse of the product, long-term contracts, and lower costs.
The income sources are strengthened by reducing turnover. To remain viable, companies and marketers must anticipate and stop consumer churn. The easiest way to achieve this is to get familiar with your clientele. Therefore, identifying behavioral trends in previous data may be a huge help here.
Using machine learning to predict churn rates from customer data enables businesses to create targeted client retention initiatives. For instance, a data science division may utilize a machine learning churn model to find high-risk customers and offer them enticing material.
Create a business case
The ultimate goal of obtaining the intended result from the ML algorithm is to identify high-risk customers so companies can reduce customer attrition. Create effective treatments to increase loyalty.
Retrieve and refine data
The following phase is data collection, which involves identifying the data sources that will power your churn prediction model. Organizations collect customer data throughout the customer journey using technologies like CRM, web and mobile analytics, sentiment analysis tools, or their databases, etc.
Converting all of this unstructured data into structured data so that your ML system can analyze it is a significant data preparation step. To create a standardized dataset for additional predictive modeling and visualization, transfer this relevant information to a platform for data processing and modification.
Design, extract, and select model features
In order to identify the characteristics that best describe the behavior patterns associated with consumer contact with a product or service, feature engineering is an essential component of the dataset preparation process. The quantifiable properties of the data points can be analyzed to determine the likelihood of churn with the help of feature engineering.
These attributes could be customer demographics, behaviors, and contextual attributes that describe additional details about a customer, such as communication preferences and previous purchasing behavior.
The variables are then standardized through feature extraction by only retaining those that convey pertinent information in the context of the business case. Data dimensions are constrained by feature extraction, which keeps the information necessary for the business case.
The advanced analytics approach to feature extraction defines the characteristics of subsets that have the greatest effect on the target variable. This results in data collection that includes the most pertinent details regarding the factors that affect attrition.
Build a predictive model
On the basis of a classification rule, ML algorithms perform binary classification to divide the characteristics of a target variable into two categories. The output of the goal variable, churn, may be categorized as either true or false. Evaluating which customers left which ones stayed is made easier by binary categorization.
Regression analysis is a statistical method used to study the relationship between a dependent variable and one or more independent variables. It is a widely used statistical tool to understand and predict the relationship between variables. For the churn prediction model, the link between the target variable and other data points that affect attrition in input data points may be determined using regression analysis based on the available data.
Random Forest is a machine learning algorithm that is used for classification and regression tasks. It is an ensemble learning method, which means that it is composed of multiple individual decision trees that work together to make a prediction. In the case of churn modeling, a random forest or a number of decision trees can be applied, depending on the size of the dataset and the variety of features.
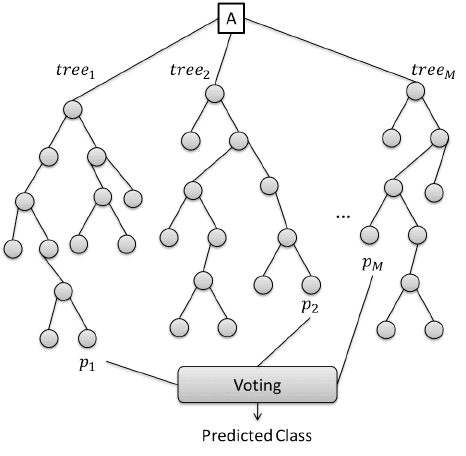
Figure 2: The Random Forest Algorithm
A decision tree is a flowchart-like tree structure that is used to make predictions based on the values of certain features. Each internal node in the tree represents a feature, and the branches represent the possible values of that feature. The leaves of the tree represent the predicted class or value. These leaves are binary in nature, the division that obtains the most votes wins.
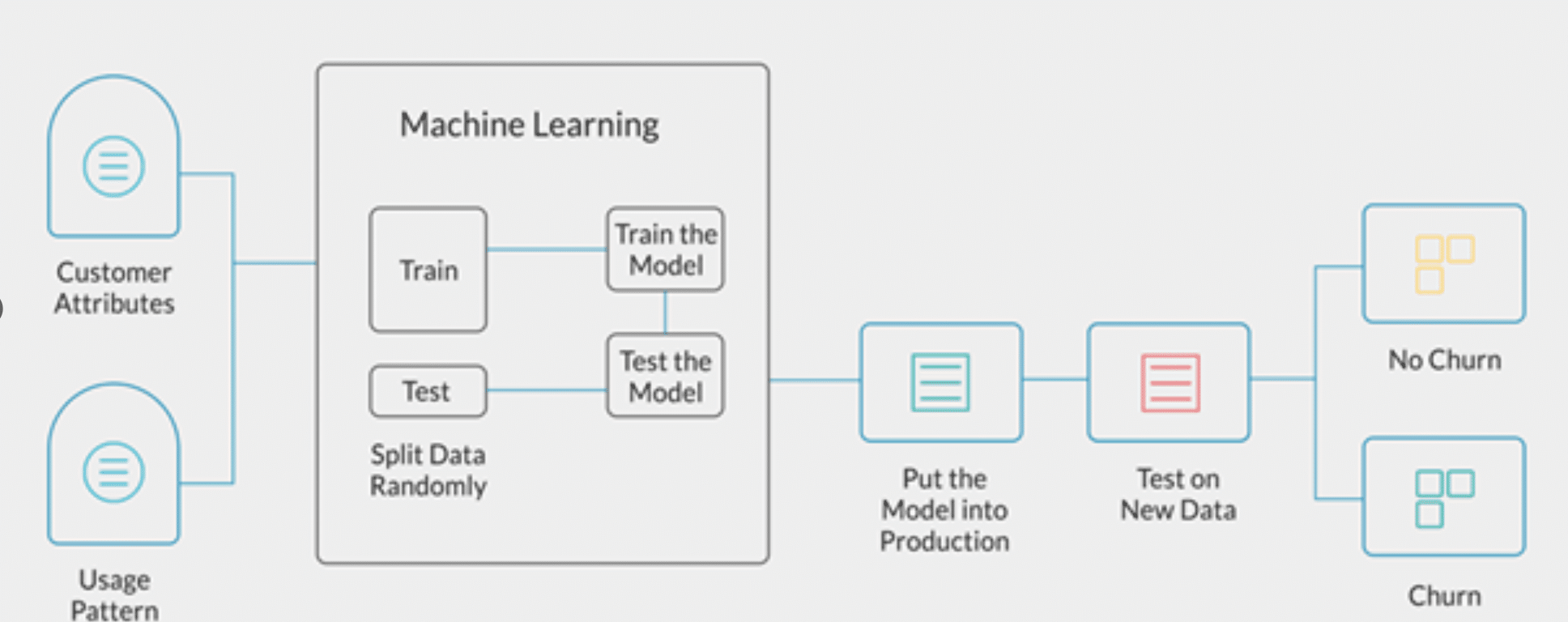
Figure 3: The Churn Prediction Model
Distribute and monitor the model
Once the model has been created, it must be connected with current software or used as the foundation for a novel program. The accuracy of the model must be closely monitored.
The performance of the classifier will be increased by testing and monitoring model performance to alter characteristics. Monitoring and testing, in the context of scenarios involving mobile applications, might entail recording consumer interactions and feedback.
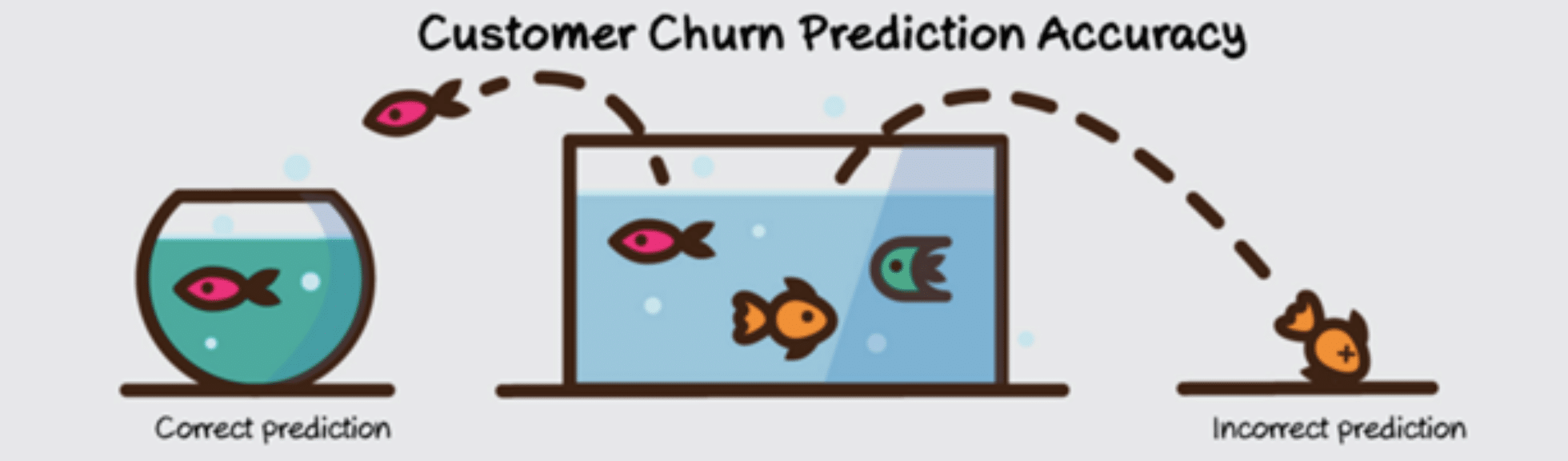
What are the application areas of churn prediction?
Reduced revenue results from high turnover rates since there are fewer consumers. Additionally, consumers who leave a business after a difficult encounter improbably leave unfavorable feedback, which will hurt the company’s reputation and make it harder to draw in new customers.
Churn findings are beneficial for firms with many customers. This translates to almost every industry. Customer churn insights are used in many sectors for several purposes. Churn prediction can be utilized in several sectors in addition to the most prevalent ones, as in the following.
Banking
The banking industry is quite unpredictable since banks must compete with one another and with other financial organizations for customers. It is difficult for banks to retain consumers because most banking products are simple to duplicate. Banks and financial institutions devote much time and money to acquiring new clients. However, Microsoft US Partner Team Blog has shown that it is less expensive to keep current customers than to get new customers. As a result, banks’ main focus should be retaining customers.
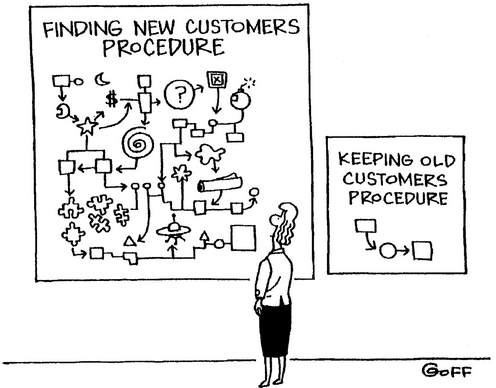
Figure 5: Finding new customers procedure
Identifying the exact worth of a potential customer’s churn would help financial institutions in their customer relationship management since the customer retention rate has a significant influence on customer lifetime value.
The banks that intend to grow their market share through client acquisition must implement customer retention measures. The best plans for a variety of loans are offered by various banks. These plans include Internet banking, mobile banking, debit cards, credit cards, savings accounts with no balances, and credit points based on customer usage. Crediting a debt to a client is a crucial activity in the group of all these facilities or programs since, in this situation, each bank must assess the customer’s ability before issuing that loan. For this reason, it is highly crucial to predict customers’ levels of credibility.
The churn prediction scores may help financial institutions decide whether to offer promotional activities. These activities can target more precise client groups with the use of customer value analysis and churn forecasts.
Due to the competitive rivalry in the banking sector, financial institutions should employ customer engagement methods even as they work to increase their market share by attracting new customers. To boost their income, banking customers may choose to use Dataroid to assess their churn tactics. Also, the likelihood of customer attrition can be decreased by Dataroid’s churn prediction approach.
Telecom
Customer loss, also known as customer defection or customer churn, refers to the rate at which customers are lost. Customer churn is frequently used by telecom businesses as a crucial business statistic to forecast the number of consumers who will switch telecom service providers.
Client satisfaction is the most efficient method of lowering customer turnover in the telecom industry due to cheap switching costs and an abundance of alternative suppliers. Utilizing the massive streams of rich telecom customer data to its fullest potential is the most efficient way to enhance the customer experience.
The approach to lowering customer turnover rates in telecom is to utilize the power of big data analytics for customer churn analysis. Scientists can extract more insightful actions by using telecom customer data to spot trends and patterns, visualize previously unobserved variable relationships, draw insightful conclusions about customer experiences, and identify the root cause of customer loss.
Customer churn prediction specifically entails the technique of allocating a likelihood of churn to each customer in the corporate database based on a forecasted correlation between that customer’s past data and its projected churning behavior. The organizations may rate their customers from most to least likely to churn with the use of Dataroid’s churn prediction technique. Customers with the highest tendency to leave get engagement initiatives.
Retail and Entertainment
When any of the following conditions are met, client churn happens in the context of digital analytics in the following ways:
- Deciding not to renew with subscription goods or services the next year after canceling them
- Refusing to reorder items and opting not to place orders in the months to come
- Continual payment default
It is essential to pay careful attention to the conversion funnel from registrations to first orders and create goals for partners in each market so that customers can see how churn is being reduced and conversion is being raised.
To effectively comprehend how customers are interacting with the platform and whether firms find value in it, churn prediction models reveal churn rates. These findings may help businesses reduce turnover.
Advances in data analytics are essential in the digital age to maintain popularity and competitiveness across expanding company trends. Businesses have started looking toward the next iteration of big data and data science solutions.
Creating a warning system that can foresee potential cancellations and launch customer loyalty initiatives is necessary. The client’s solutions might affect the client’s viewpoint and reduce turnover. Dataroid assists customers in defining the measures to lower the churn rate by assisting them in performing effective churn analytics.
Key Takeaways
Churn prediction enables businesses to lower the churn rate by industries. The most widely applied sectors can be listed as the following ones:
- Digital Banking
- Telecom
- Retail and/or Entertainment
Having a well-structured dataset is crucial for accurate churn prediction, but it can be challenging to achieve this on your own. Digital analytics tools can play a significant role in helping businesses to generate structured churn prediction results. Real-time and big data analytics tools, in particular, offer powerful capabilities that can help to identify patterns and trends quickly.
Digital analytics tools as Dataroid can provide insights into customer behavior and engagement, enabling businesses to make data-driven decisions. Dataroid’s churn prediction solution offer a comprehensive approach to customer retention. Dataroid’s AI-powered solution can help businesses identify the factors that contribute to churn, predict customer behavior, and take proactive steps to reduce attrition rates. By utilizing tools like Dataroid’s churn prediction solution, businesses can improve their ability to retain customers and ultimately grow their revenue.
Sign up to drive your business with the power of data
Drive your digital growth
Schedule a demo today to learn more on how we can help you unleash the potential of digital using Dataroid.