Anomaly Detection: Identifying Critical Issues to Reduce Revenue Losses
- Reading Time: 7 minutes
In the contemporary age, the amount of information produced is greater than ever. As sales promotions ingest enormous amounts of data, the information collected might be scattered or redundant, raising questions about the accuracy of the information they include.
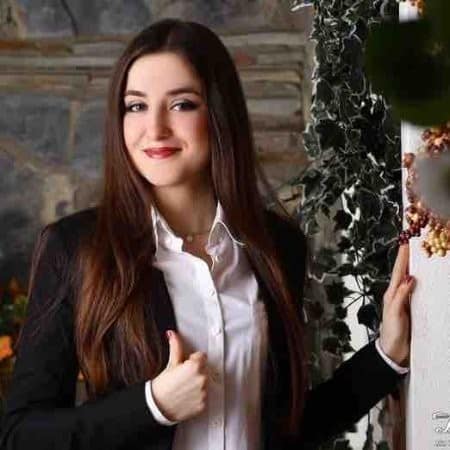
Revenue departments yearn for useful knowledge and the best course of action, yet they are overwhelmed by fragmented data. To obtain precise, fast, and efficient judgments, the data squads need to swiftly extract meaningful information from enormous data sets.
What is anomaly detection?
It is possible to identify abnormal activity in the data source. A data source anomaly is a sudden shift or departure from the predicted trend. Outliers signal aberrant behavior, indicating what is causing the problem or not occurring appropriately. Although odd points aren’t always positive or negative, businesses should be aware of them to decide whether they need to take action or not.
Companies produce large numbers of observations daily, but most of this important data is lost or ignored. Feature extraction methods are becoming highly popular in the corporate sector as they help expedite and enhance activities to create a more foreseeable future.
Sign up to drive your business with the power of data
Why is anomaly detection important?
Detecting outliers can be challenging to recognize. Following are some indicators of a system or application’s abnormal flow of actions.
- Increase in the cost of a campaign
- Lower/higher purchasing activity than expected
- Target audience differing noticeably from average in performance
- Unusually low visitors to a web page/application, indicating a problem
- Drastic change in single sessions ratios
- Increment in page exits
- Decrement/increment in user engagement metrics
What data types are used for anomaly detection?
Advanced technologies for minimizing uncertainty include machine learning anti-fraud solutions, which optimize the challenging task of abnormality detection. Artificial intelligence systems can identify customer data patterns and extremely subtle, frequently hidden occurrences that might signify misrepresentation. 
Large-scaled data collections may be handled via anomaly detection with machine learning, which compares several factors in real-time to assess the likelihood of anomalous activities or transactions. 
Technology has improved over time in simultaneously tracking and processing. The following types of information can be collected to be analyzed for outlier behavior: 
- Location/region data 
- Time-series data 
- Device/hardware data 
- Purchase-related revenue data 
- Transaction volume data 
In addition to processing more financial data than rule-based approaches, ML can help in intrusion detection systems. Reducing the number of verification steps that slow down the purchasing process and eliminating false positives are made possible by clever systems that monitor user behaviors.
How does anomaly detection work?
Visual Exploration
To discover unusual activity, input or industry experts create graphical analysis. Finding solutions with the correct data visualization frequently requires previous business expertise and creative thinking. Lower-dimensional charts using sophisticated representations, such as those produced using principal components, may be used to access high-dimensional data.
Supervised Learning
Individuals with business expertise in a certain sector are used in supervised methods to classify a set of information sets as typical or unusual. A data scientist uses labeled data to create models using machine learning that forecast abnormality in unlabeled data.
Semi-Supervised Learning
The advantages of the preceding two approaches are combined in semi-supervised methods for identifying anomalies. Data scientists can use unsupervised methodologies to automate feature learning and work with unstructured data. For this reason, by integrating it with human supervision in a semi-supervised manner, they can track the trends discovered by the models. This typically helps to improve the accuracy of the model’s estimations.
Unsupervised Learning
Unsupervised models are constructed using unlabeled data to forecast existing data points. Since the algorithm is designed to suit regular data, few aberrant metrics settle out.
Time Series
Oddities are discovered using time series algorithms that identify patterns, seasonalities, and levels in time series data. Excessive deviation of new information from the forecast can be due to a model malfunction or an abnormality.
Auto-encoders
Modern generative models and auto-encoder algorithms quickly identify abnormalities and take appropriate action. An auto-encoder algorithm can forecast abnormalities from transactional and sensor reading inputs.
Clustering 
Every data item may be classified by aggregating with the help of data scientists into one of the numerous recognized or undiscovered categories; instances that do not conform to an established category can be considered outliers.
What are the application areas of anomaly detection?
Artificial intelligence techniques have effectively automated hardware and software processes through computerized systems and automation technology. They have also helped deduce meaning from massive volumes of domain-generated textual and quantitative data.
Furthermore, machine learning has evolved to take a proactive role in decision-making assistance by developing tools that use descriptions and knowledge discovery to anticipate possible results. Anomaly detection is utilized in several sectors in addition to the most prevalent ones, as in the following.
Digital Analytics
Example Case Study: Log anomaly detection
Log data saves essential system operational data. Digital platform solutions need to pinpoint and distinguish computer system failures. Each data center processes massive lines of log records per day. Traditionally, companies would manually analyze logs using keyword search and rule matching. However, the expansion of log volume has rendered proper evaluation ineffective.
For this reason, it is crucial to investigate anomaly detection using log analysis. The log messages are gathered from real-world applications. Organizations must employ artificial intelligence techniques to evaluate log data from unsuccessful runs to assist companies in performing efficient problem diagnosis.
Finance
Example Case Study: Detection of excessive amounts of money transfer via cards or bank accounts
In the domain of payment and finance, fraud detection is crucial. Recent mainstream banking firms utilize real-time outlier detection in audits to stop losses in their tracks. To keep ahead of cybercriminals, organizations may either purchase whole anomaly detection systems or build them from scratch.
An enterprise requires a sophisticated anomaly detection system that uses ML (machine learning) to monitor and correlate several complex indicators with variable degrees of variation while poring over millions of data points per second to maintain real-time reactivity. Many members of the internal audit team and the financial department have limited expertise in statistical skill sets, including process and data mining and tabular analytics. Furthermore, they might be unable to track, analyze, or identify information vulnerability indicators.
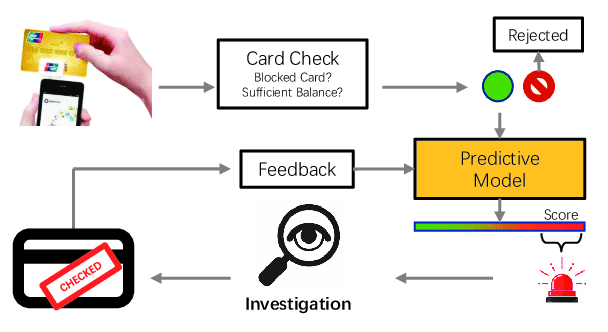
Figure 1: An anomaly detection system for cards
Cybersecurity
Example Case Study: Intrusion detection
Recent years have seen a significant increase in the interest in identifying abnormalities across a wide range of security domains. The number of network intrusions is often a relatively tiny percentage of all network activity in network security. While abnormalities are uncommon, they are increasingly essential in these cases related to other events, making it crucial to identify them.
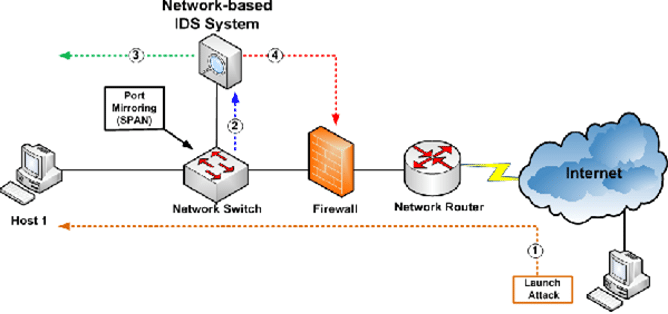
Figure 2: A network-based anomaly detection system
Insurance
Example Case Study: Detection of false insurer activity
In the healthcare system, insurance-based anomalous activity is widespread and has cost businesses significant amounts of money in payouts to criminals. Health insurers must detect false statements to guarantee that no payments are made to false identities. In the last decade, many companies have invested significantly in artificial intelligence to develop machine learning models to recognize insurance fraud.
Medical and insurance companies may create supervised, unsupervised, and semi-supervised models to lower the possibility of fraudulent activity for every request received using algorithms and outlier detection tools.
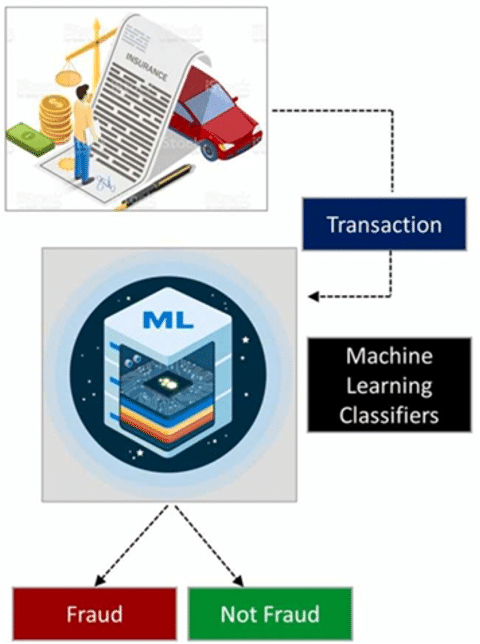
Manufacturing
Example Case Study: Diagnosis of the problematic unit
Various organizations continually use deep learning models to monitor manufactured components’ sensor data. Experts identify and fix any problematic units as they arise as the model evaluates new information.Several top producers are beginning to adopt neural network models, since manually inspecting for errors and abnormalities can cost them more time and cash. By employing a machine learning approach, businesses may track and immediately identify any unexpected events utilizing sensor data from manufactured units.
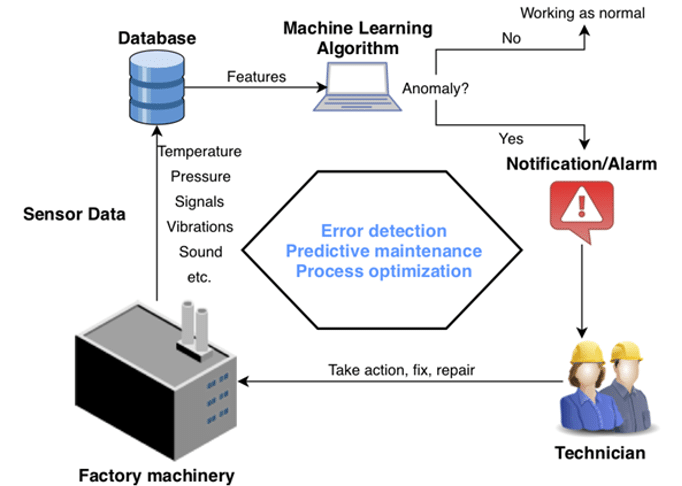
Figure 4: A manufacturing anomaly detection system
How can Dataroid help with anomaly detection?
Dataroid is a digital analytics and customer interaction technology that uses data to improve each customer encounter and enhance their journey. Considering Dataroid as a digital and predictive analytics solution, it is simpler to incorporate outlier detection methods into the organizations’ massive data volumes.
Key Takeaways
The data collected by enterprises today is more significant than ever. Companies shall be able to observe behaviors, identify abnormalities, and prevent substantial company losses, including malfunctioning infrastructure, fraud, and faults.
Organizations may gain valuable insights, improve efficiency, and compete better in the information age by spotting abnormalities in statistics. Corporations may employ model-based machine learning with advanced analytics technology to define and track user input and identify unusual activity for improved business impacts. Anomaly detection will likely continue to gain acceptance in various usage areas, such as security and clinical applications.
Sign up to drive your business with the power of data
Drive your digital growth
Schedule a demo today to learn more on how we can help you unleash the potential of digital using Dataroid.